Junhong Xu
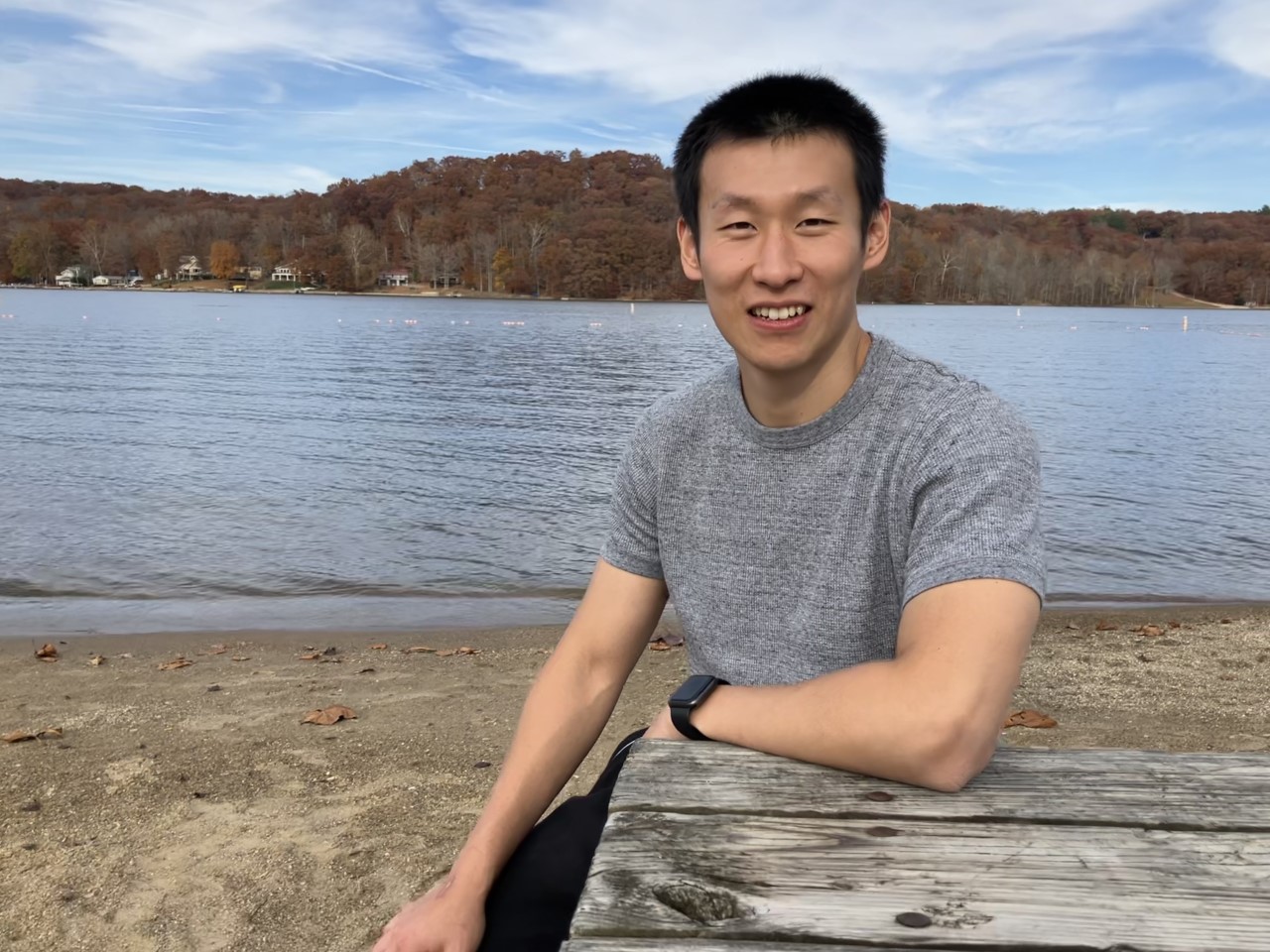
About Me
I am currently at Nuro.ai working on safe reinforcement learning methods and generative models for addressing challenging, safety-critical problems in autonomous driving. Prior to Nuro.ai, I obtained my PhD from Indiana University, Bloomington, where I worked with Lantao Liu in Vehicle Autonomy and Intelligence Lab.
My research focuses on designing methods that enhance the safety and efficiency of robotic systems at deployment time. To achieve this goal, during my doctoral research, I focused on leveraging model uncertainty to improve deployment time robustness. I utilized potential model inaccuracies to develop planning algorithms that adopt conservative behaviors in high-uncertainty regions. Towards the end of my PhD, I also worked on generating diverse and trainable environments for reinforcement learning, aiming for deployment time generalization over environment distributions.
News
-
October, 2024 One paper got accepted by CoRL 2024, enabling generating realistic and challenging environments for training generalizable robot navigation policies.
-
October, 2024 Our work on using the diffusion model to imagine unknown regions to provide more informative context for the downstream planner is accepted by IROS 2024.
-
July, 2024 We published a new blog post on how Nuro.ai combines safe RL and imitation learning for self-driving.
-
April, 2024 Boundary-Aware Value Function Generation for Safe Stochastic Motion Planning got accepted by the International Journal of Robotics Research (IJRR). This work combines mesh-based and meshless function approximators to accelerate the computation of solving the second-order Hamilton-Jacobi Bellman (HJB) equation while not sacrificing the strict boundary conditions on critical state dimensions.
-
January, 2024 One paper got accepted by the International Journal of Robotics Research (IJRR), proposing a novel kernel-based approach for solving stochastic optimal control problems and its application to autonomous navigation on unstructured terrains.
-
October, 2023 A new arXiv paper on enhancing the sampling-based MPC algorithm (MPPI) with uncertainty propogation.
-
October, 2023 Our work on combining informative prior policies trained by goal-conditioned RL with the bounded-rational game-theoretic framework is accepted by IROS 2023.
-
August, 2023 I successfully defended my PhD dissertation titled “Robust Motion Planning and Control for Autonomous Robots Under Uncertainty”.
-
October, 2022 One paper on learning a causal model of the robot’s dynamics is accepted by ICRA 2023.
-
September, 2022 Our paper Decision-Making Among Bounded Rational Agents on explicit modeling the computational limits in multi-agent motion planning using information-theoretic bounded rationality is accepted by DARS 2022.